MALEGRA - Multimodal Signal Processing and Machine Learning on Graphs
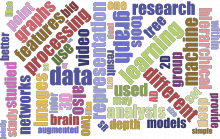
Type | Start | End |
---|---|---|
National | Jan 2017 | Jun 2021 |
Responsible | URL |
---|---|
Javier Ruiz-Hidalgo / Xavier Giró |
Reference
MALEGRA, TEC2016-75976-R, financed by the Spanish Ministerio de Economía, Industria y Competitividad and the European Regional Development Fund (ERDF)
Description
The goal of this project is to study and develop tools combining graph signal representation and processing ideas with machine learning technology. These tools will be used in the context of applications where the size and/or the heterogeneity of the data represent challenges of the Big Data era. The development of technologies related to the capture, storage, search, distribution, transfer, analysis and visualization of ever growing heterogeneous datasets entails tremendous difficulties. At the same time, these difficulties open new opportunities and this development has become a major trend in the field of Information and Communication Technology. The research performed in this project targets applications such as multi-view representations, video analysis, remote sensing for earth monitoring, person identification, health monitoring, medical imaging, genomics, etc.
The project has 4 major objectives. The first two objectives concentrate most of the development of theoretical and basic tools within the project. Within them, we will investigate the creation, analysis, segmentation, filtering and merging of graph structures of heterogeneous multimodal data and on the combination of these ideas with machine learning techniques. This combination with machine learning will be used for several different purposes. In particular, to provide a classification decision, to learn a mapping or a model to be used in a data processing architecture, to learn features that outperform handcrafted equivalents or to aggregate several features to create a signal to be further processed.
The last two objectives of the project focus on the application of the techniques and tools developed in the first two objectives in complex challenges that deal with big and heterogeneous data. In particular, these techniques and tools will be used to study the identification of persons in broadcast TV programs, the optimal encoding of depth maps in multi-view plus depth representations, the radiometric estimation and object detection in SAR and PolSAR images, the classification of multispectral and hyperspectral images, the understanding of brain changes during the evolution of Alzheimers disease, the inference of gene regulatory networks and the segmentation, tracking, indexing and super-resolution of multimodal video sequences.
Publications
Wav2Pix: Speech-conditioned Face Generation using Generative Adversarial Networks. In: ICASSP. ICASSP. Brighton, UK: IEEE; 2019.![]() |
.
Depth Estimation and Semantic Segmentation from a Single RGB Image Using a Hybrid Convolutional Neural Network. Sensors. 2019 ;19(8).![]() |
.
Correspondence matching in unorganized 3D point clouds using Convolutional Neural Networks. Image and Vision Computing. 2019 ;83-84.![]() |
.
Retinal lesions segmentation using CNNs and adversarial training. In: International Symposium on Biomedical Imaging (ISBI 2019). International Symposium on Biomedical Imaging (ISBI 2019). ; 2019. | .
CNN-based bacilli detection in sputum samples for tuberculosis diagnosis. In: International Symposium on Biomedical Imaging (ISBI 2019). International Symposium on Biomedical Imaging (ISBI 2019). ; 2019. | .
Standardized Assessment of Automatic Segmentation of White Matter Hyperintensities; Results of the WMH Segmentation Challenge. IEEE Transactions on Medical Imaging. 2019 . |
One Shot Learning for Generic Instance Segmentation in RGBD Videos. In: International Conference on Computer Vision, Theory and Applications. International Conference on Computer Vision, Theory and Applications. Prague: SciTePress; 2019.![]() |
.
Assessing Knee OA Severity with CNN attention-based end-to-end architectures. In: International Conference on Medical Imaging with Deep Learning (MIDL) 2019. International Conference on Medical Imaging with Deep Learning (MIDL) 2019. London, United Kingdom: JMLR; 2019.![]() |
.
Study of early stages of Alzheimer’s disease using magnetic resonance imaging . Signal Theory and Communications. 2019 ;PhD. | .
Multiresolution co-clustering for uncalibrated multiview segmentation. Signal Processing: Image Communication. 2019 .![]() |
.
Pages
Collaborators
Javier Ruiz Hidalgo | Associate Professor | j.ruiz@upc.edu |
Xavier Giró | Associate Professor | xavier.giro@upc.edu |
Ferran Marqués | Professor | ferran.marques@upc.edu |
Albert Oliveras | Associate Professor | albert@tsc.upc.edu |
Philippe Salembier | Professor | philippe.salembier@upc.edu |
Elisa Sayrol | Associate Professor | elisa.sayrol@upc.edu |
Josep R. Casas | Associate Professor | josep.ramon.casas@upc.edu |
Veronica Vilaplana | Associate Professor | veronica.vilaplana@upc.edu |
Montse Pardàs | Professor | montse.pardas@upc.edu |
Marc Maceira | PhD Candidate | marc.maceira@upc.edu |
Xiao Lin | PhD Candidate | xiao.lin@upc.edu |
Alba Pujol | PhD Candidate | alba.pujol@upc.edu |
Adrià Casamitjana | PhD Candidate | adria.casamitjana@upc.edu |
Albert Gil Moreno | Software Engineer | albert.gil@upc.edu |