DIORAMA: Data-centric AI for medical imaging
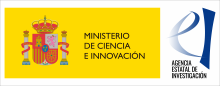
Type | Start | End |
---|---|---|
National | Sep 2024 | Dec 2027 |
Responsible | URL |
---|---|
Verónica Vilaplana & Philippe Salembier |
Reference
AEI ID: PID2023-148614OB-I00
UPC ID: J-03278
Acknowledgements for publications:
PID2023-148614OB-I00 research project funded by MICIU/AEI /10.13039/501100011033 and by FEDER, EU.
Logo for presentations and posters
Description
The field of machine learning has witnessed remarkable advancements in recent years, with the development of powerful model architectures, primarily based on deep learning techniques, capable of achieving exceptional performance on a wide range of tasks. However, the successful application of these models in real-world settings, particularly in the healthcare domain, often faces significant challenges. One of the primary limitations is the reliance on high-quality datasets for training or fine-tuning these models. In many medical applications, obtaining such datasets is an arduous task due to the scarcity of data, data quality issues, class imbalance and the need for expert annotation.
To address these challenges, a new paradigm has emerged in machine learning, known as Data-Centric Artificial Intelligence (DCAI). DCAI proposes a shift from a model-centric approach, focusing on designing and optimizing complex models, to a data-centric perspective, emphasizing the crucial role of data in determining the performance and effectiveness of AI models.
DCAI is guided by three principles: (i) data quality, or ensuring the accuracy, completeness, consistency and relevance of training data; (ii) data reliability, which includes identifying and mitigating biases, errors, and inconsistencies; and (iii) data comprehension, which involves understanding the underlying data distributions and relationships. Adopting a data-centric approach offers several benefits including improved model performance, reduced model bias and enhanced model explainability.
Furthermore, integrating clinical experts into the data-centric AI process is crucial for success in healthcare applications. Their domain knowledge and insights can help in identifying data quality issues, providing clinical context for annotating and labeling data, selecting appropriate data augmentation techniques, and interpreting model outputs.
The DIORAMA project aims to explore the application of data-centric AI to address specific medical problems in three domains: histopathology imaging, magnetic resonance imaging, and thermal imaging. Our approach will involve (i) exploring theoretical and generic tools for data curation and learning on limited datasets, (ii) investigating generative models as a form of data augmentation for training machine learning models and (iii) applying data-centric AI techniques to address specific medical tasks in each domain.
We will use data-centric AI to address three distinct medical conditions: cancer, Alzheimer’s disease and diabetes. Each research line is supported by ongoing collaborations with healthcare institutions: breast cancer with the eight major hospitals associated with the Institut Català de la Salut, prostate cancer with the Vall d’Hebron Institut de Recerca, Alzheimer’s disease with the Barcelona Brain Research Center, and diabetes with Institut Universitari d’Investigació en Atenció Primària (IDIAP Jordi Gol) and the Institut d'Investigació Biomèdica de Bellvitge (IDIBELL)
We expect that the proposed techniques will contribute to the gradual incorporation of complex machine learning and deep learning decision support algorithms into clinical practice, and to understand and address the limitations that these tools may have in such challenging environments.
Publications
Layer-Wise Training of Graph Neural Networks With Self-Supervised Learning. IEEE Transactions on Neural Networks and Learning Systems,. In Press . | .
Comparative Analysis and Interpretability of Survival Models for Melanoma Prognosis. Computers in Biology and Medicine. 2025 ;190. | .
Neuroimaging-derived biological brain age and its associations with glial reactivity and synaptic dysfunction cerebrospinal fluid biomarkers. Molecular psychiatry. 2025 . | .
Towards a multicentric open DigitAL PatHology assIstant beNchmark: Initial Results from the DALPHIN Study. In: United States & Canadian Academy of Pathology 114th Annual Meeting (USCAP 2025). United States & Canadian Academy of Pathology 114th Annual Meeting (USCAP 2025). Boston, USA: Laboratory Investigation, Volume 105, Issue 3, Supplement, 2025, 103609, ISSN 0023-6837; 2025. | .
Towards a multicentric open DigitAL PatHology assIstant beNchmark: Initial Results from the DALPHIN Study. In: United States & Canadian Academy of Pathology 114th Annual Meeting (USCAP 2025). United States & Canadian Academy of Pathology 114th Annual Meeting (USCAP 2025). Boston, USA: Laboratory Investigation, Volume 105, Issue 3, Supplement, 2025, 103609, ISSN 0023-6837; 2025. | .
Breast Cancer Molecular Subtyping from H&E Whole Slide Images using Foundation Models and Transformers. In: Artificial Intelligence and Imaging for Diagnostic and Treatment Challenges in Breast Care. Vol. 15451. Artificial Intelligence and Imaging for Diagnostic and Treatment Challenges in Breast Care. Springer; 2025. | .
Identifying brain ageing trajectories using variational autoencoders. In: PRedictive Intelligence in MEdicine. Vol. 15155. PRedictive Intelligence in MEdicine. Springer International Publishing; 2025. | .
Identifying brain ageing trajectories using variational autoencoders with regression model in neuroimaging data stratified by sex and validated against dementia-related risk factors. In: 7th International Workshop on PRedictive Intelligence in MEdicine, MICCAI 2024. 7th International Workshop on PRedictive Intelligence in MEdicine, MICCAI 2024. ; 2024. | .
Breast Cancer Molecular Subtyping from H&E Whole Slide Images using Foundation Models and Transformers. In: Deep Breast Workshop on AI and Imaging for Diagnostic and Treatment Challenges in Breast Care, MICCAI 2024. Deep Breast Workshop on AI and Imaging for Diagnostic and Treatment Challenges in Breast Care, MICCAI 2024. ; 2024. | .
Collaborators
Josep R. Casas | Associate Professor | josep.ramon.casas@upc.edu |
Ferran Marqués | Professor | ferran.marques@upc.edu |
Montse Pardàs | Professor | montse.pardas@upc.edu |
Philippe Salembier | Professor | philippe.salembier@upc.edu |
Veronica Vilaplana | Associate Professor | veronica.vilaplana@upc.edu |
Oscar Pina | PhD Candidate | oscar.pina@upc.edu |
Claudia Giardina | PhD Candidate | claudia.giardina@upc.edu |
Lauren Jimenez | PhD Candidate | lauren.jimenez@upc.edu |
Maria Ysern | PhD Candidate | maria.ysern@upc.edu |