Graph based Dynamic Segmentation of Generic Objects in 3D
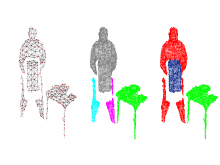
Resource Type | Date |
---|---|
Results | 2016-05-03 |
Description
We propose a novel 3D segmentation method for RBGD stream data to deal with 3D object segmentation task in a generic scenario with frequent object interactions. It mainly contributes in two aspects, while being generic and not requiring initialization: firstly, a novel tree structure representation for the point cloud of the scene is proposed. Then, a dynamic manangement mechanism for connected component splits and merges exploits the tree structure representation. The following 3 videos show the experiments results.
Experiment 1 (comparison experiments on 3 sequences):
In this experiment, we compare the segmentation performance of our approach with the approach proposed in [1] on three sequences oriented to the scene of object interaction. Sequence 1 contains a scenario of a human hand rolling a green ball forward and then backward with the fingers. Sequence 2 involves a robot arm grasping a paper roll and moving it to a new position. Sequence 3 describes a scenario in which a human hand enters and leaves the scene, displacing the objects rapidly, leaving little or no overlap of corresponding segments.
In this video, the segmentation result of each frame in these sequences is shown as: the color image in this frame (left), the segmentation result from our approach for this frame (middle) and the segmentation result from [1] (right).
Experiment 2 (Quanlitative and quantitative results on 4 sequences from Human Manipulation Dataset [2]):
In this experiment, we show the segmentation result of our approach on 4 sequences from Human Manipulation Dataset [2]. These four sequences focus on the scene that a human manipulates the objects such as juice box, mug and milk box on the table. They vary from single attachment to multi-attachment, low motion to higher motion, double attached objects to multiple attached objects.
In this video, the experiment result of each frame in these four sequences is shown as: the color image in this frame (left), the segmentation results of our approach shown in the component level (middle), in which each component is painted with a random color and the segmentation result of our approach shown in the object level (right), in which the color of each object interprets its correspondence along the sequence and the detected object interactions (presented with a white line connecting two interacting objects in the image).
Experiment 3 (Extra experiment on dataset recorded by ourslef)
In this experiment, we show the segmentation result of 4 sequences recorded by ourself. These four sequences focus on the scene that a full human body interacts with objects such as box, handcart, dog etc.
In this video, the experiment result of each frame in these four sequences is shown as: the color image in this frame (left), the segmentation results of our approach shown in the component level (middle), in which each component is painted with a random color and the segmentation result of our approach shown in the object level (right), in which the color of each object interprets its correspondence along the sequence.
People involved
Xiao Lin | PhD Candidate |
Montse Pardàs | Professor |
Josep R. Casas | Associate Professor |
Related Publications
“3D Point Cloud Video Segmentation Based on Interaction Analysis”, in ECCV 2016: Computer Vision – ECCV 2016 Workshops, Amsterdam, 2016, vol. III, 9915 vol., pp. 821 - 835.![]() |
,
“Graph based Dynamic Segmentation of Generic Objects in 3D”, in CVPR SUNw: Scene Understanding Workshop, Las Vegas, US, 2016.![]() |
,