Body pose dataset
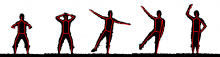
Resource Type | Date |
---|---|
Dataset | 2015-09-22 |
Description
Introduction
The dataset has 12 recorded subjects performing 10 different standstill body poses of different complexity. It has been recorded using a Kinect camera and both the image and depth information is provided. A groundtruth consisting of an event register which associates body poses global identifiers to the sequence frame intervals in which they were being performed. Also, an articulated body model is given per frame (for body skeleton estimation) providing a set of body articulation positions. The articulation points stored are: head, neck, right shoulder, right elbow, right hand, left shoulder, left elbow, left hand, right knee, right hip, right foot, left knee, left hip and left foot. The dataset can be used for academia purposes only (not commercially).
Download
README
Subject00.zip
Subject01.zip
Subject02.zip
Subject03.zip
Subject04.zip
Subject05.zip
Subject06.zip
Subject07.zip
Subject08.zip
Subject09.zip
Subject10.zip
Subject11.zip
Groundtruth and body articulated model
A common representation of the human body pose is an articulated model involving joints that connect every rigid part. Due to its simplicity and having high coherence with pose description it is often used in human body estimation problems.
Figure 4.1: NITE articulated body model representation with labels on each joint.
In our context, we use a set of joint positions ai related to body articulations as the body pose physical groundtruth. To retrieve articulation positions for each frame we applied during capture a body skeleton tracker from the OpenNI/NITE library. We consider this tracker sufficient accurate to become the dataset physical groundtruth.
Related to gestures, an identification number is given to each pose-gesture. In order to establish a groundtruth GT we modified the recording program to manually register gestures in real-time with the keyboard. Consequently, for each frame a scalar number identificator g is bound as the pose-gesture groundtruth. Additionally, associated to the capture an events list is saved indicating the frame intervals in which every gesture is performed. These annotations are used later in the detector to skip transitions between gestures.
GT = { ai ∈ R3 | i = 1. . #joints , g ∈ G | card(G) = #gestures}
Dataset Description
The dataset has 2 sequences called: Basic and Advanced. Each one has 5 pose-gestures. In Figure 2 a representation of the articulated model is given for every proposed gesture. Basic sequence is designed with clear gestures and keeping symmetry. On the other hand, Advanced present more disturbed gestures.
Figure 2: Proposed data set body gestures with respective articulated skeleton. First row of gestures corresponds to the Basic sequence. Bottom row corresponds to the Advanced sequence.
Every subject will perform both sequences and a repetition of each one. A total of 4 recordings per subject captured and are labeled as A,B,C,D corresponding to sequences Basic, Basic (repetition), Advanced, Advanced(repetition) respectively.
Having 12 subjects the total recordings composing the dataset is 12 subjects x 4 recordings = 48 recordings.
Figure 3: the dataset folder hierarchy with description tags.
Finally, the dataset is organized in a hierarchy of folders described in Figure 3. For every recording the following data is stored per captured frame: depth image, color image and groundtruth. Afterwards, point clouds are extracted from depth images (Figure 4).
Figure 4: The 3 dataset formats. Color image (left), depth image (middle) and point cloud (right).
People involved
Javier Ruiz Hidalgo | Associate Professor |
Josep R. Casas | Associate Professor |