3D Point Cloud Segmentation using a Fully Connected Conditional Random Field
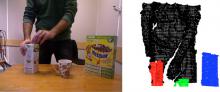
Resource Type | Date |
---|---|
Results | 2017-03-05 |
Description
3D Point Cloud Segmentation using a Fully Connected Conditional Random Field
- Supplementary Material For EUSIPCO 17'
Traditional image segmentation methods working with low level image features are usually difficult to adapt for higher level tasks, such as object recognition and scene understanding. Object segmentation emerges as a new challenge in this research field. It aims at obtaining more meaningful segments related to semantic objects in the scene by analyzing a combination of different information. 3D point cloud data obtained from consumer depth sensors has been exploited to tackle many computer vision problems due to its richer information about the geometry of 3D scenes compared to 2D images. Meanwhile, new challenges have also emerged as the depth information is usually noisy, sparse and unorganized.
We present a novel point cloud segmentation approach for segmenting interacting objects in a stream of point clouds by exploiting spatio-temporal coherence. We pose the problem as an energy minimization task in a fully connected conditional random field (CRF) with the energy function defined based on both current and previous information. We compare different methods and prove the better segmentation performance and robustness of the proposed approach in sequences with over 2k frames.
1. Qualitative examples of segmentation result from CRF and super-voxel level fully connected CRF (S-FC-CRF) based method on sequential frames
These examples show that the crf based method segmentation sticks to the thiner part of a point cloud while the fully connected crf based method provides better segmentation on the boundary due to the fact that fully connected structure makes the graph shape less critical for segmentation.
Color Image CRF S-FC-CRF
2. Segmentation results shown in a video
Segmentation result of a point cloud sequence in the video below:
Figure 1. Segmentation result of a point cloud sequence. Different objects are labelled in different colors.
View in YouTube (https://youtu.be/Ca6UWIUCx20)
People involved
Xiao Lin | PhD Candidate |
Montse Pardàs | Professor |
Josep R. Casas | Associate Professor |